Introduction by Blog Coordinator Darnell Lattal, Ph.D.
Janet has agreed to produce a series of 3 blogs on generative AI and its implications for the ABAI Behavior Analysis in Organizations site. Each stands alone in its rationale and importance. They provide a tutorial for those of us thinking about our science, our commitment to improving the human condition, and the emerging methods and tools of generative AI. They address the potential impact on understanding the science of behavior analysis in the larger community, particularly regarding knowledge accuracy or misinformation. She asks us to consider our personal accountability for tracking and responding to misleading AI data generation. The series will help us better understand the opportunities and difficulties that lie ahead, ones that are likely to fundamentally affect our practices. She also describes the powerful impact of AI when correctly linked to the knowledge content of the science of behavior to help us all better serve humankind. In a nutshell, an important as well as a good read.
Navigating the Ethical Terrain of Generative AI in Behavior Analysis: A Three-Part Series
Janet S. Twyman
We are witnessing a world shifting under our feet. Throughout our collective careers as behavior analysts, we have relied on a wide range of tools and instrumentation (see Lattal & Yoshioka, 2017), beginning with the use of relay racks and operant chambers and continuing into the modern era of personal computers, wearable devices, and smartphones. Yet the new field of Generative Artificial Intelligence holds the greatest potential for altering behavior analytic research and practice. With its accessibility, ease of use, and ability to generate original content, generative AI has the potential to fundamentally change how we work and learn.
Powered by machine learning algorithms that identify patterns in data, generative AI is a technology that is capable of generating new content (e.g., text, images, speech, audio, computer code) in response to requests from users (often called “prompts) (Webster, n.d.). Trained on an enormous reference database of examples, this technology is characterized by its capacity to learn, adapt, and complete multiple and complex tasks across domains. Its use has spread from computer science labs and tech companies into universities, public and private corporations, schools, hospitals, clinics, and other settings where behavior analysts work.
Generative AI is already occasioning radical change. For behavior analysts, the tools based on this technology could serve as an intelligent assistant that could serve as a personal tutor, help with exam prep, process volumes of research or treatment data, detect or predict trends across volumes of data, read visual displays, translate audio or text, serve as a personal assistant, write draft task analyses, or perhaps even aid in customizing or summarizing treatment plans (also see Cox & Jennings, 2023). Yet the integration and application of generative AI into behavior analysis is not just about harnessing cutting-edge technology; it’s about how behavior analysts ethically leverage such technology to create positive change.
As with any powerful technology, there are ethical considerations around its responsible development and use that we must proactively navigate, giving rise to questions such as:
- How can we ensure client well-being and privacy when algorithms process sensitive data?
- Who bears responsibility for the conduct of an AI system designed to support ABA therapy delivery?
- What safeguards are needed to prevent misuse or misinterpretation of AI-generated outcomes?
- How do we uphold the dignity of individuals receiving ABA therapy augmented by AI?
Ethics in the clinical practice of ABA involves making decisions that promote the welfare and dignity of clients, maintaining professional conduct, and adhering to established standards. It has always been the backbone of behavior analytic practice. The underlying theme of ethical AI use in behavior analysis resonates with the humanistic emphasis on empathy and understanding, aligning with B. F. Skinner’s humanistic roots (Newman et al., 1996). The behavior analytic approach centers on respecting and fostering individual development, selecting goals and procedures due to their importance to the person and to society, and emphasizing personal progress (Baer et al., 1968). Our first and foremost consideration in using generative AI should be its alignment with the humanistic values at the core of our profession. The technology, with its profound ability to generate original content, analyze complex behaviors, and predict outcomes, must be implemented to reflect sensitivity to the unique needs of individuals. That perspective will remain constant as we consider the ethical use of AI.
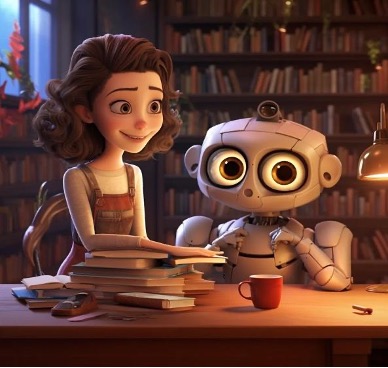
Each post in this three-part series will contain a list of suggested guidelines to ponder. Part 1, this blog, covers universal considerations important for the ethical use of generative AI across many fields. Part 2 covers more specific concerns directly relevant to behavior analysts and those doing similar research and practice. [Readers interested in a more in-depth analysis of this topic are encouraged to read the recent publication, “Starting the Conversation Around the Ethical Use of Artificial Intelligence in Applied Behavior Analysis” by Jennings and Cox (2023)], appearing in Behavior Analysis in Practice). Part 3 addresses AI in organizations and systems and how this technology could affect organizational dynamics and ethics within a profession.
Part 1: Universal Considerations in the Ethical Use of Generative AI
Transparent Informed Consent
As behavior analysts increasingly use generative AI in their research and practice, informed consent becomes even more critical. Our duty is to ensure that our consumers and clients (and their caregivers) are fully aware that AI is being used, what data are being collected, how those data are being used, what types of predictions or analyses the AI might generate, and the implications of the use of AI. Educating others about how AI is used in analysis and treatment, describing benefits and limitations will foster transparency and encourage active participation in behavior analytic implementation.
An Example: In conducting a university study using AI to analyze student learning patterns, provide detailed consent forms explaining what data is being fed to the AI system and what might be expected as outputs.
Privacy and Data Security
Behavior analysts are charged with protecting sensitive information. Handling sensitive behavioral data generated by AI systems requires stringent measures to protect confidentiality and ensure data security. Even with anonymized data, there may be a risk (albeit small) of re-identification; thus, strong data security measures are an ethical necessity. We must engage in the usual safeguards of secure data storage, restricted access, encrypted data transfers, and proper destroying records (see Motti & Berkovsky, 2022), and we must be especially vigilant in removing all personally identifiable information. This protects privacy and prevents generative AI from inadvertently revealing identifying details in future outputs.
Additionally, Generative AI systems continue to learn from the information provided, adapting and refining their knowledge to improve performance. Any data entered has the potential to shape or influence future outputs. (It’s also why consent around how data is used for AI training is so important.) Hence, we need to be highly cautious and conservative about the data we enter into a generative AI tool, as the information has the potential to be remembered and reflected in unpredictable future generations of outputs. Obviously, fully anonymized data with consent is vital for the ethical use of such systems.
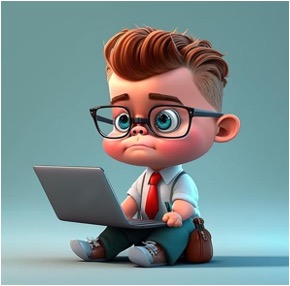
An Example:
A behavior analyst uses a natural language processing AI system to analyze therapy session transcripts for a group of clients with language delays to identify verbal patterns and customize treatment plans. Before inputting any transcripts, all potentially identifying information like client names, specific locations mentioned, or identifiable personal details are redacted manually and through automated scrubbing. The cleaned transcripts are securely uploaded to the cloud-based AI system, leveraging encryption, access controls, and data deletion policies meeting regulated standards. Resulting AI-generated insights are stored only in the clinician’s internal patient files not disseminated further without individual consent. Clients also formally consent to using redacted transcripts for AI analysis to drive therapeutic improvements while preserving autonomy and confidentiality.
Legal Compliance and Regulation
Our use of AI must fit within the framework of existing laws, regulations, and policies, whether they are national, state, or from the organizations and institutions in which we work. While the legal landscape surrounding AI is evolving (much like the technology itself), behavior analysts must stay informed about data protection and privacy laws, ensuring that our use of AI advances our objectives and fully aligns with legal standards. These are a few of the resources (linked) that can help behavior analysts stay informed about legal compliance and regulation in AI:
US Federal AI Governance
Blueprint for an AI Bill of Rights
Executive Order on Safe, Secure, and Trustworthy Artificial Intelligence
UNESCO: Artificial Intelligence in Education
UNICEF: Policy guidance on AI for children
Artificial Intelligence and the Future of Teaching and Learning
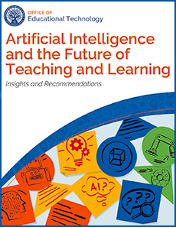
Recognizing and Addressing Bias
Bias is harmful and insidious, permeating all human systems—and AI is not exempt. Generative AI models reflect biases encoded in their training data and algorithms, intentionally or not (Jo, 2023). As AI researcher Joy Buolamwini (2023) astutely states, “computers reflect both the aspirations and the limitations of the people who create them.” The powerful use of algorithms based on human-created and human-centric data heightens the concerns we already have around discrimination, profiling, inequity, loss of control over information, and the misuse of personal information.
In behavior analysis, the potential for bias can have profound implications, from erroneous behavioral predictions to prejudiced intervention strategies. Our role involves vigilant and ongoing searching for biases, actively seeking to eliminate them, and confirming that the AI’s use, data, procedures, and products are fair and equitable for all individuals. It is essential to recognize and address potential biases in these systems to prevent discriminatory practices, ensure fairness, and regularly audit AI systems to ensure they do not perpetuate or amplify discriminatory practices.
An Example: In a project for a corporate client, an AI tool could be less accurate with data from certain demographic groups, requiring retraining of the algorithm to ensure fair and unbiased analysis.
Two More Examples:
1) “A behavior analyst uses a generative AI tool to track facial expressions and vocal tones during therapy sessions to provide automated feedback to practitioners on client engagement levels. However, upon reviewing the AI’s performance, it is found to accurately assess vocal tone changes but under-detect facial engagement cues for persons of color due to facial recognition data limitations.
To address this, the analysts first notify the AI developer, calling for expanded and more representative facial recognition training data. In the meantime, the analysts mitigate the automated vocal tone feedback and disable use of the facial recognition capabilities until equal efficacy across skin tones is verified through third-party auditing. They continue manually gauging engagement for all clients rather than rely on the AI’s imbalanced perceptions.
The analysts responsibly avoided perpetuating discriminatory practices by identifying limitations in the AI’s training data that manifested as detection biases and addressing the root issue by calling for expanded data diversity. Their choice to incur a temporary loss of functionality protects client interest and scientific integrity while spurring positive changes.”
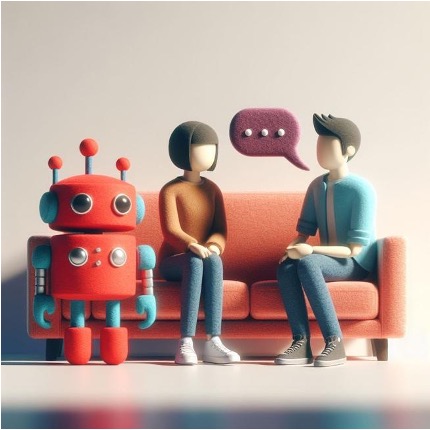
2) “A public school is piloting a generative AI chatbot to provide basic counseling and mental health support to students seeking help. However, analysis reveals the chatbot is displaying gender bias – dismissing concerns shared by female students at a much higher rate than male counterparts.
In response, the school discontinues chatbot use immediately, pending improvements to avoid perpetuating discriminatory tendencies. They require the AI developer team to incorporate stronger debiasing efforts, including augmented training data with better representation of perspectives from women and minority communities.
Additionally, all chatbot counseling interactions are recorded, reviewed, and manually changed if biases emerge prior to re-deployment. Student focus groups also continually provide feedback on chatbot performance. Through multi-pronged mitigation and monitoring efforts centered on inclusiveness, the risks of biased AI counseling advice are minimized while still providing this supplement to student support capabilities.
The keys are recognizing limitations quickly, ceasing usage until deficiencies are addressed, mandating equitable development processes, and ongoing human oversight of automation. This upholds fairness and goodwill towards all student groups rather than simply ignoring or exploiting an imbalanced AI tool.”
Accountability
Who should be held accountable in an entirely unwanted scenario in which an AI-generated finding, recommendation, or intervention leads to unforeseen harm or negative outcomes? Determining accountability when using AI tools poses complex challenges. If a product or intervention, even partially drafted by generative AI, contributes to undesirable outcomes, multiple stakeholders shoulder responsibility: the AI developer, the behavior analyst user, administrators, executives, and other decision-makers who support its use.
Such a scenario should raise further questions about the level of understanding and control practitioners have over the AI tools they use and motivate behavior analysts to gain a working understanding of the AI tools they use. To better understand how these tools work, developers of the tools we use must maximize algorithmic transparency. Shared accountability encourages corporate, clinical, and research cultures to implement AI thoughtfully, responsibly, and for the common good.
An Example: A behavioral health organization implements a machine learning system to review intervention data and adjust plans automatically. However, flaws in the AI system lead to recommending less than optimal reinforcement schedules for some patients, leading to a slight increase in disruptive behavior.
Upon review, one could determine that the Generative AI developer is at fault for failing to train extensively on reinforcement schedules before deployment. The administrators who purchased the software ignored practitioner objections about efficacy and the need to safeguard implementation. The behavior analysts who directly relied on AI-adjusted plans without adequate review need to be required to undergo re-training on behavioral principles and responsible AI usage.
Part 1 Conclusion
When using generative AI in research or practice, our professional and ethical obligation is to restrict the dissemination of sensitive information, thoroughly vet the results it gives us, and ensure we have control over AI-generated outputs. AI should work in tandem with our human intellect and behavior analytic knowledge base to generate beneficial results. Our responsibility is to ensure that generative AI is used in a conscientious and secure manner. Using deliberate foresight to guide the ethical use of generative AI can greatly sharpen our analytical capacities while improving human welfare, equality, and opportunity.
Part 1 Suggestions for Using Generative AI in Behavior Analysis:
Obtain/Maintain Informed Consent
– Secure informed consent by providing comprehensive, understandable information about the AI’s role and impact.
– Regularly update consent forms to reflect evolving AI functionalities.
– Create plans for educating clients about AI, including its role, benefits, and limitations in their treatment or analysis.
– Encourage client participation in decisions related to AI use in their behavioral analysis or treatment.
Prioritize Transparency
– Clearly communicate how Generative AI is used, its capabilities, and limitations.
– Ensure that stakeholders understand the implications of AI-generated data and predictions.
Maintain Data Privacy and Security
– Anonymize data where possible, encrypt and store data securely, and restrict access to authorized persons only.
– Regularly update your security protocols and conduct audits.
– Ask yourself, “If these data were about me, would I feel safe sharing it?”
Comply with Legal Regulations
– Understand how current laws and regulations impact the use of Generative AI in general, within certain fields or professions, and with specific populations.
– Recognize that the legal landscape regarding AI, data protection, and privacy laws is evolving.
Validate and Verify AI Outputs
– Regularly evaluate the accuracy and reliability of AI-generated analyses and predictions, especially in critical decision-making processes.
– Compare outputs across sources, including original research or other publications.
Address AI Bias
– Continuously monitor your AI outputs for bias.
– If bias is detected, promptly implement corrective measures (including disregarding the output, revising the output, and, whenever possible, notifying the developer/source).
– Request documentation and communication on how the algorithms are designed, what training data is used, and what safeguards are in place to combat bias.
Shared Accountability
– Use generative AI systems that enable human users to understand how or why the tools arrived at certain outputs. The logic should not be opaque or too complex to grasp.
– Use generative AI systems with thorough documentation explaining their development, strengths, limitations, and safeguards to manage risks.
– Design protocols for auditing algorithms to assess for unfair biases, errors, and risks.
Footnotes
1. While I am the sole author of this post, several tools were used to support its text and image creation, content modification, and the final draft. These include Anthropic’s Claude, Learneo’s Quillbot, Microsoft’s Copilot, Microsoft Image Creator from Designer, and Open AI’s Chat GPT. My role included topic inspiration, content generation, organization, quality control and synthesis of AI-generated content, and tone, voice, and style writer.
2. This example was generated in entirety by Anthropic’s Claude AI, when given the prompt: Please provide “An Example” given this topic and description: (Insert Recognizing and Addressing Bias and the accompanying text.)
3. This example was generated in its entirety by Anthropic’s Claude AI, when given the prompt: Please provide another example on the same topic.
4. Algorithmic transparency refers to the concept of ensuring AI and machine learning systems are understandable and interpretable rather than opaque “black boxes” (Claude, 2023)
5. This example was generated by Anthropic’s Claude AI, when given the prompt: Please provide “An Example” given this topic and description: (Insert Accountability and the accompanying text). Underlined text indicates the author’s modifications to the output.
6. This list was generated with the assistance of Open AI’s Chat GPT, Anthropic’s Claude, and Microsoft’s Copilot, using the prompt, “Please provide a bulleted list of recommendations concerning the ethical use of AI, using topic headers.” The results from the three tools were synthesized and revised by the author.
Guest Author: Janet Twyman
Dr. Janet S. Twyman is an educational consultant, Chief Learning Scientist, and founder of blast: A Learning Sciences Company. With a diverse professional background as a preschool and special education teacher, principal, university professor, researcher, and instructional designer, Dr. Twyman has dedicated her career to promoting effective learning technologies that drive equity and individual and system change. She has presented to or worked with over 80 education systems, states, and countries and was an invited speaker on technologies for diverse learners and settings at the United Nations. She formerly served as Director of Innovation and Technology for the U.S. Dept. of Education Center on Innovations in Learning. Dr. Twyman has published extensively on instructional design, virtual/remote learning, evidence-based innovations in education, and systems to produce meaningful differences in learners’ lives. In 2008, she served as the President of the Association for Behavior Analysis International. In 2014, she was named an ABAI Fellow. Her distinguished contributions to educational research and practice have been honored with the Wing Award for Evidence-based Education and the American Psychological Association Division 25 Fred S. Keller Behavioral Education Award.
References
Anthropic. (2023). Claude 2.1. [Large Language Model]. https://claude.ai/chats
Baer, D. M., Wolf, M. M., & Risley, T. R. (1968). Some current dimensions of applied behavior analysis. Journal of Applied Behavior Analysis, 1(1), 91–97.
Buolamwini, J. (2023). Unmasking AI: My Mission to Protect What Is Human in a World of Machines. New York: Random House.
Cox, D. J., & Jennings, A. M. (2023). The Promises and Possibilities of Artificial Intelligence to in the Delivery of Behavior Analytic Services. Behavior Analysis in Practice, 1-14.
Generative AI. (2023). In Merriam-Webster.com. Retrieved from https://www.merriam-webster.com/dictionary/generative%20AI
Jennings, A. M., & Cox, D. J. (2023). Starting the Conversation Around the Ethical Use of Artificial Intelligence in Applied Behavior Analysis. Behavior Analysis in Practice, 1-16.
Jo, A. (2023). The promise and peril of generative AI. Nature, 614(1), 214-216. Retrieved from https://www.nature.com/articles/d41586-023-00340-6
Lattal, K. A. & Yoshioka, M. (2017). Instrumentation in Behavior Analysis. Mexican Journal of Behavior Analysis, 43(2), 133-136. doi.org/10.5514/rmac.v43.i2.62309
Microsoft. (2023). Copilot. Large language model]. https://adoption.microsoft.com/en-us/copilot/
Motti, V. G., & Berkovsky, S. (2022). Healthcare Privacy. In Modern Socio-Technical Perspectives on Privacy (pp. 203-231). Cham: Springer International Publishing.
Newman, B., Reinecke, D. R., & Kurtz, A. L. (1996). Why be moral: Humanist and behavioral perspectives. The Behavior Analyst, 19, 273-280.
OpenAI. (2023). ChatGPT-4 Turbo [Large language model]. https://chat.openai.com/chat
Image Credits
Image 1 – Woman and robot in library: Midjourney – Not subject to copyright
Image 2 – Male working on laptop: Image by Alexandra_Koch from Pixabay (ai-generated-7772469_1280)
Image 3 – Cover of ebook, “Artificial Intelligence and the Future of Teaching and Learning: Insights and Recommendations” from The U.S. Department of Education Office of Educational Technology, found at https://tech.ed.gov/ai-future-of-teaching-and-learning/
Image 4 – AI image generated by the author using the prompt “Two people sitting on a couch talking, with a cute robot standing nearby listening, Style: abstract surrealism minimal felt cloth sewn art, 3d animated, colorful” on Bing AI